A successful project management system must include or integrate with an adaptive and effective quality management system. ISO (the International Organization for Standardization, 9000:2015, https://www.iso.org/obp/ui/#iso:std:iso:9000:ed-4:v1:en) defines a quality management system (QMS) as a set of well-defined policies, processes and procedures required for planning and execution in design, development, production, and services in business areas that can impact the organization’s ability to meet customer requirements. Some people generically refer to a group of documents as a QMS. But specifically, it refers to the entire system – the documents just describe it. An effective QMS enables the organization to identify, measure, control, and improve the various core business processes that will ultimately lead to improved business performance. To date there are few, if any, QMS that integrate the tracking and monitoring of physical services activities with advanced analytics to deliver real-time insights and actions in support of optimized field services for infrastructure systems.
Quality assurance (QA) and quality control (QC) are two key aspects of a quality management system. While some quality assurance and quality control activities are interrelated, they are inherently different. Typically, QA activities and responsibilities cover virtually all of the quality system in one fashion or another, while QC is a subset of the QA activities.
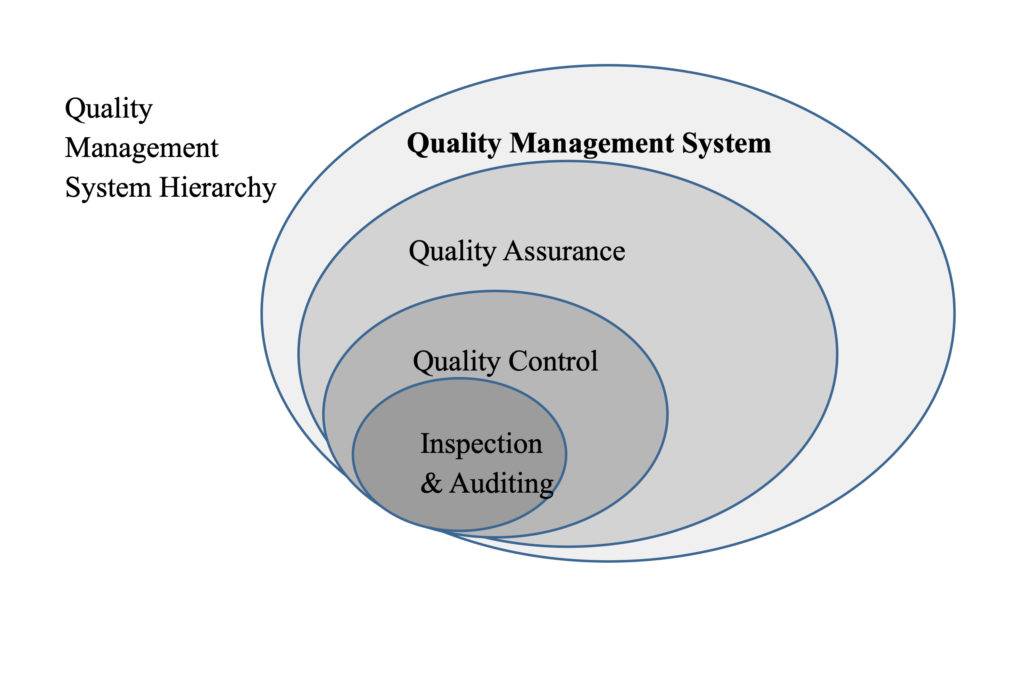
QA is defined as “part of QMS focused on providing confidence that quality requirements will be fulfilled” (https://asq.org/quality-resources/quality-assurance-vs-control). The confidence provided by quality assurance is twofold—internally to management and externally to customers. As a subset of QA, QC is focused on fulfilling quality requirements. While quality assurance relates to how a process is performed or how a product is made, quality control is more the inspection or verification aspect of quality management. Auditing and inspection can be another important part of the quality assurance function, defined as comparing actual conditions with requirements and/or expectations, and reporting those results to management and/or other organizations.
Like manufacturing systems, infrastructure systems and related field services have started to deploy technology enabled capabilities. These can support the effective implementation of advanced quality management functions.
The first key technology enabler is the Internet of Things (IoT) with integrated sensors and controls, where each major component of an infrastructure system is connected to the internet, monitored and/or controlled, and data is collected. Unfortunately, IoT deployments produce massive amounts of real-time data, which can be challenging for field service providers to effectively use without some advanced tools.
The second key technology enabler is analytic solutions. These solutions can provide more accurate and faster insights. Initially, raw data from IoT and modern infrastructure systems were not ready for analytics. Unfortunately, analysts must clean, extract, transfer and load (ETL) data, seriously constraining the QMS system’s ability to provide real-time controls and/or predictive services. To address this constraint, automated data validation or checking technology must be integrated into the system, between the IoT components and analytics engine, to support collection of clean real-time data that can be correlated and effectively used in computer algorithms and analytics to produce accurate insights, responses, and reports.
One approach to adding analytics to the management and servicing of infrastructure systems is to mash up or stack QMS solutions with analytic solutions. Note, however, that this approach will produce a loosely coupled platform unable to support real-time controls or service activities. While this mashup may support some level of dashboarding and reporting on operations, it would not deliver the advanced and/or differentiated capabilities expected by today’s customers.
The ultimate goal is to integrate the analytics engine with the QMS so completely that neither the service provider nor the customer will even know they are using advanced analytic techniques and machine learning. This tight integration of QMS and analytic solutions will help users be more productive and accurate in their decision making and service delivery. This integration will also enable automation of reports, guides, and alerts. Actionable information will appear in context to help make better quality decisions without having to jump to a different analytic user interface. Quality investigations and decisions will ultimately be streamlined to improve the quality of any field service delivery solution.